Creating Ai-First Workflows
OUR MISSION: General AI
WoRld-CLass Research
VISION: Redefining the FUTURE OF WORK
Our vision is to pioneer an AI-first open-source platform in Europe, dedicated to democratizing the latest advancements in AI.
Including breakthroughs like GPT-4 and other proprietary technologies. We firmly believe in catalyzing Europe’s resurgence as a global leader in the AI domain by fostering an open market.
REsearch & Development

Building applications with large language models at the core using our Symbolic AI
facilitates the integration of classical and differentiable programming in Python.
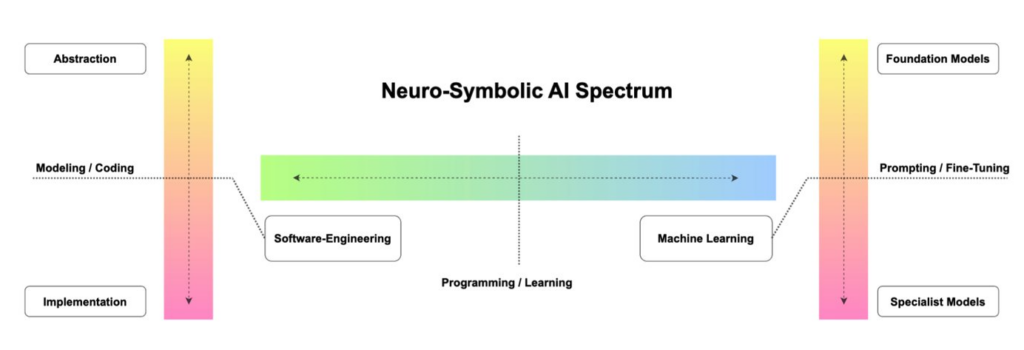
We develped an innovative framework designed to orchestrate operations through task-specific prompts. Embracing a divide-and-conquer strategy, we dissect complex challenges into smaller, more digestible components. This method ensures that each operation tackles a more straightforward aspect of the task. Upon reintegration, these segmented operations collectively address the larger, intricate problem. Furthermore, the foundational principles of our framework facilitate a fluid transition between differentiable and classical programming models. This integration capitalizes on the strengths of both paradigms, offering a robust and versatile solution.
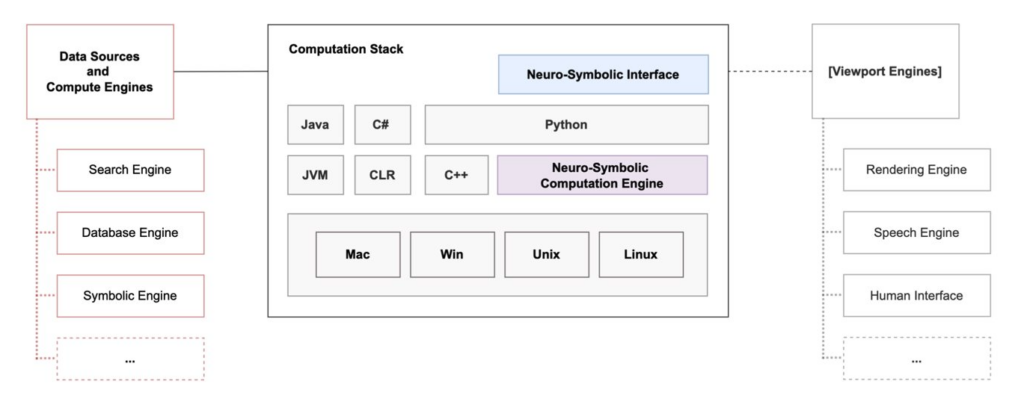
Our system thrives on the principle that as long as objectives are articulable in natural language, LLMs become powerful tools for neuro-symbolic computations. We’ve engineered operations that adeptly manipulate these symbols to create new, meaningful constructs. Each symbol is interpreted as a discrete statement, and by amalgamating multiple statements, we construct comprehensive logical expressions.

In the realm of software evolution, our framework represents a significant leap, bridging the gap between classical programming (Software 1.0) and differentiable programming (Software 2.0). This transition occurs along two critical dimensions: firstly, moving seamlessly between traditional and differentiable programming paradigms; and secondly, shifting effortlessly between generalist and specialist models. This dual adaptability forms the cornerstone of what we term in-context realization of applications, a concept central to Software 3.0. In this new era, applications are not static; they are dynamic entities capable of evolving over time. This fluidity and adaptability mark a radical departure from conventional software design, reflecting a more organic and responsive approach to software development.
Videos
Testimonials
What Others SAY ABOUT US
Building applications with LLMs at the core using our SymbolicAI
framework facilitates the integration of classical and differentiable programming in Python.
Sepp Hochreiter
“Happy to share this recent work on ‘A Neuro-Symbolic Perspective on Large Language Models (LLMs)’. It bridges the gap between classical programming (Software 1.0) and differentiable programming (Software 2.0) by combining the power of LLMs and a symbolic approach.”
Alexander Morrise
“Working on SymbolicAI now – great work! This will be foundational!”
Thomas Wildberger
“Very nice. I love it. Totally confident that SW developers in a few years will learn a development paradigm like that in their training.”
We specialize in developing generative AI solutions that combine neuro-symbolic systems, blending symbolic reasoning’s precision with the adaptability of neural networks. Our approach, focused on advanced large language models (LLMs), excels in processing and translating complex documents across diverse sectors. This innovative blend of technology facilitates the simplification of intricate texts, making them accessible and comprehensible to a broader audience.
GENERATE ENTIRE SOLUTIONS
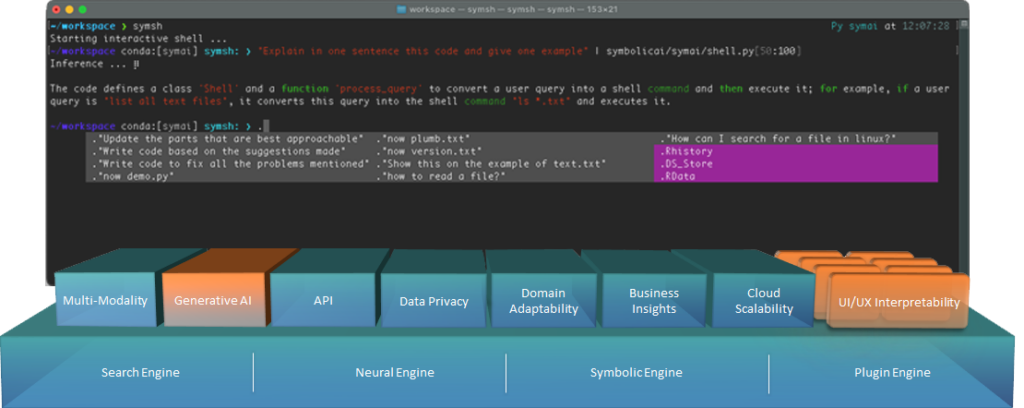
Pricing
AI Platform
For more information on collaboration opportunities, please feel free to contact us.
Enterprise Plan
On Request
Our Expertise
15+ Years of Experience in Research & Development
We are a team o highly qualified researchers, developers and business leaders, with a global network of industry leading contacts.
Thomas
Neumayer
Business Strategy – Marketing
Markus Hofmarcher
Machine Learning
Senior Researcher
Claudiu Leoveanu-Condrei
Machine Learning
Senior Researcher
Robin
Goupil
Senior Fullstack
Developer
Marius-Constantin Dinu
Machine Learning
Research Director
John Chong Min Tan
Machine Learning Researcher
Stefan
Dorninger
Finance
Legal
ADVISORY BOARD
Sepp Hochreiter
We are honored to introduce Univ.-Prof. Dr. Sepp Hochreiter, an esteemed computer scientist renowned for his pioneering contributions to machine learning and artificial intelligence. He has made significant strides in the research field, notably co-developing the Long Short-Term Memory (LSTM) neural network architecture, and with numerous accolades under his belt, including the German AI Innovation Award for 2023, he continues to influence the advancement of AI with proficiency and foresight.
Andreas Windisch
We are honored to introduce Dr. Andreas Windisch, a theoretical physicist with a profound dedication to artificial intelligence and high-performance computing. As a passionate researcher and AI Austria Chief Science Officer, Dr. Windisch applies his expertise to advance cutting-edge research and foster collaborative innovation across varied disciplines and AI ecosystems.
OUR NETWORK
Ready To Start New Project With ExtensityAI?
Are you excited to embark on a new venture with ExtensityAI? Whether you’re looking to integrate cutting-edge AI solutions into your existing systems or start a fresh project harnessing the power of artificial intelligence, we’re here to bring your vision to life. Our team of experts is ready to collaborate with you, offering tailored solutions that align with your specific needs. Let’s create something remarkable together. Reach out to us now and take the first step towards transforming your ideas into reality!
ExtensityAI
Copyright © 2024. All rights reserved.